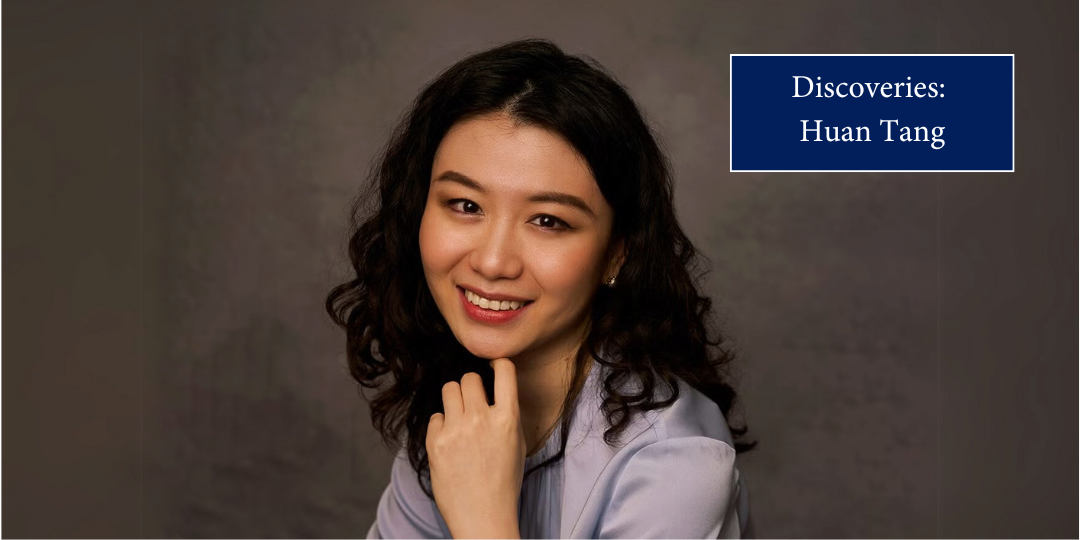
What is the scope of data-sharing across firms, and what are the economic implications? In her study, “Data as a Networked Asset,” supported by the Stevens Center for Innovation in Finance, Huan Tang, assistant professor of finance at Wharton, uncovers a hidden network of inter-firm data flows – revealing that companies are connected not only by products or supply chains, but by the information they exchange. This research shows that this data network influences how firms perform, how they respond to shocks, and how they are valued in the market. Professor Tang explains the financial and economic implications of data as a networked asset in the conversation below.
What is the relevance of your research for investors, companies, and policymakers?
Huan Tang: Our paper is motivated by the unprecedented scale of the data economy. With smartphones in every pocket and tracking technologies like cookies embedded in websites and apps, companies today can collect and exchange data on users in real time—at massive scale.
This shift is exemplified by the rise of data aggregators like Oracle and Acxiom, who’ve quietly built detailed profiles on billions of individuals by pulling data from a wide range of sources—what we browse and buy, where we go, and even who we interact with.
As firms become increasingly connected through data sharing, it’s important to understand how they use data, how they exchange it, and what the broader consequences of this sharing are. Our research sheds light on this by uncovering the data-sharing network among firms, offering insights for companies seeking data-driven growth, for investors evaluating firm value, and for policymakers designing data privacy laws that could reshape the structure of the data economy in ways we don’t yet fully grasp.
Your research identifies data sharing as an economic linkage between firms. How does data link firms, and how does this differ from other industry linkages?
Huan Tang: We show that data-based connections between firms are largely distinct from traditional linkages like supply-chain relationships or product-market overlap. For example, Amazon’s top data-connected peers include General Motors, American Express, Vodafone, and Morgan Stanley—firms that span very different industries.
This suggests that we’ve uncovered a novel type of economic linkage. Rather than being connected by physical inputs or shared markets, these firms are linked by the way they use and benefit from each other’s data. This opens a new lens for thinking about how firms interact and create value in the digital economy.
You find that companies connected through shared data often move together in the stock market — even when they don’t operate in the same industry. Why is that, and how does this shape our understanding of financial markets?
Huan Tang: This is at the heart of our study. Firms use data as a productive asset—to understand customers, target products, and drive demand. But data isn’t isolated within each firm; it’s part of a broader data-sharing network. One company’s data can help another make better decisions.
Take Amazon again: data from GM about car purchases, financing, or connected vehicles could help Amazon predict demand for car accessories or stock inventory in regions where electric vehicle adoption is surging. So, when one firm improves its data, its peers also benefit. We call this phenomenon data externalities.
This interconnected nature of data explains why stock returns of data-linked firms can move together, even when their core businesses are seemingly unrelated. It reveals a hidden layer of economic interdependence that investors should pay attention to.
What does your research reveal about shock propagation in the digital economy, when firms share data on such a large scale?
Huan Tang: In line with the return co-movement pattern, we find that shocks—such as a cyberattack—can ripple through the data network. A disruption at one firm doesn’t stay contained; it can affect its data-connected peers, particularly when the firm is central in the network.
This has important implications. It suggests that the digital economy has its own channels for shock propagation. Just like financial contagion in banking, data-sharing can become a pathway for spreading disruption, even across firms that aren’t directly competing or transacting.
You conclude that data sharing firms make product design decisions that exhibit herding. What do you mean by herding, and what is the impact on these firms’ industries?
Huan Tang: Herding means that firms tend to move together in their product design choices—such as offering free features to attract users and accumulate data, or switching to paid features to monetize the customer base.
This choice involves a tradeoff: focusing on user engagement now helps grow the firm’s data assets, while monetization brings in short-term cash flow but may slow down data growth. What we find is that firms’ decisions are shaped by what their data peers are doing.
Because of positive data externalities, if some firms offer free features and ramp up data collection, others benefit from the improved insights without bearing the full cost. This encourages them to also lean toward engagement-focused strategies. Conversely, if peers start prioritizing monetization, others may follow.
This herding effect means that shifts in firms’ product design—often influenced by external factors like privacy regulations—can cascade across the network. Today, many digital products are free. But changes in policy or consumer attitudes could tip the balance toward paid features, potentially transforming the entire digital landscape.
How can future research build upon your findings?
Huan Tang: People often say, “Data is the new oil.” That’s not far off—as data becomes a core input in production. But unlike physical capital, data is intangible, difficult to measure, and behaves in unique ways.
Our paper is a first step in measuring and characterizing data as a networked asset. The data network we construct, along with measures of centrality and connectedness, could help researchers and practitioners better understand how data-driven firms are valued and how shocks propagate in the data economy.
Future work could build on this by asking: How do firms choose their data peers? What types of network structures lead to the most productivity in the data economy? And how can we design policies that balance consumer privacy with innovation and growth in the data economy?
Learn more about Professor Huan Tang.
This research was supported by the Stevens Center for Innovation in Finance.
View the study, “Data as a Networked Asset.”